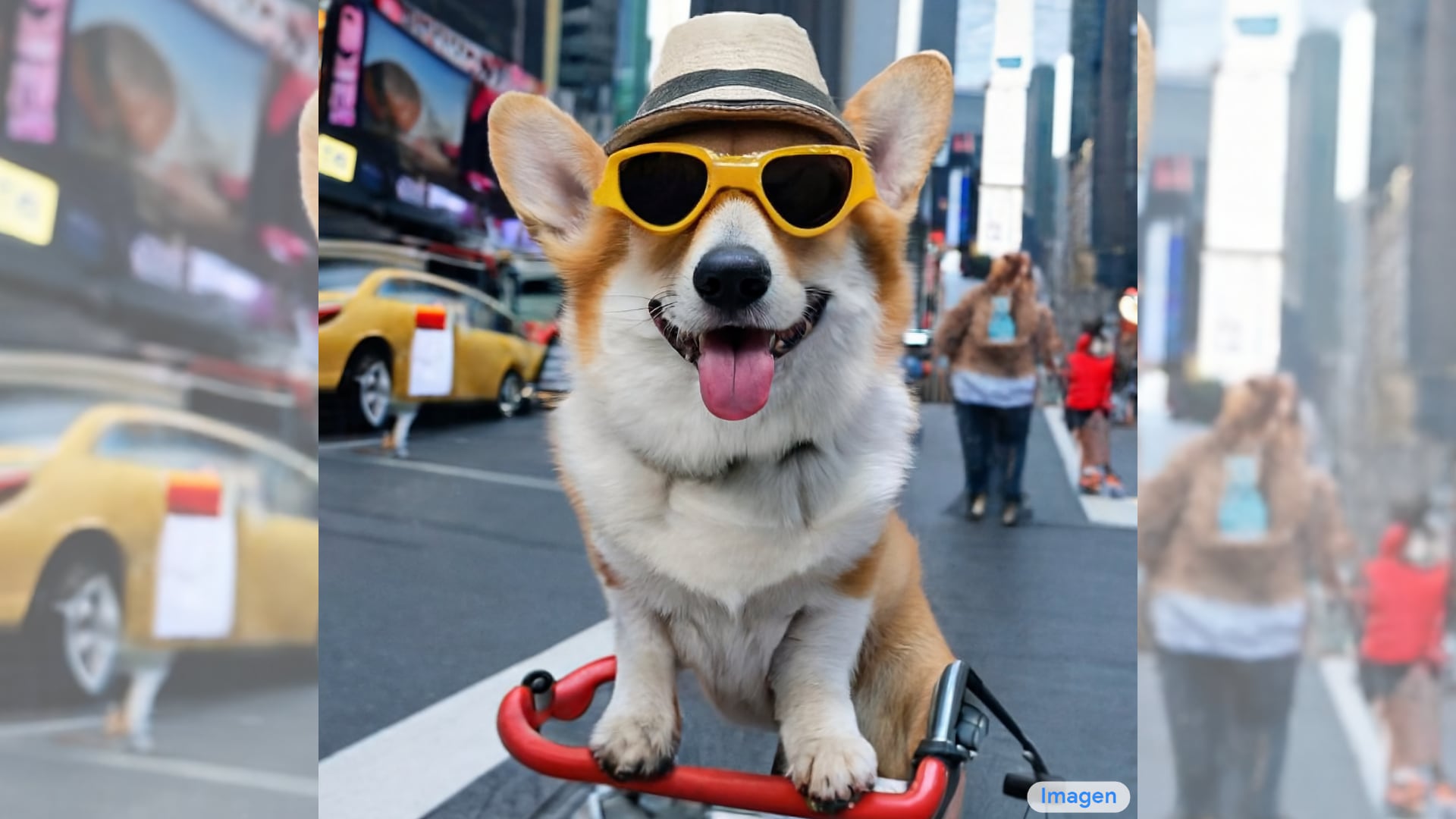
With the likes of Dall-e 2, Midjourney, and Stable Diffusion already part of the cultural fabric, just over six months after Google’s Project Imagen was first released it can now already produce video.
Various eminent scientists, including Einstein and Feynman, have been quoted, or perhaps misquoted, as having made statements to the effect that anything which can’t be explained simply isn’t sufficiently well understood. Neither of them is still around to witness the burgeoning success of machine learning and artificial intelligence, which is a shame. Not only might they both have had the intellectual chops to fully comprehend what’s going on, and give us a digestible breakdown, but it’d have been good to hear Feynman’s typically pithy reaction to widespread excitement over a video of an elephant in a party hat.
The video in question is part of a presentation about Google’s project Imagen (sic) Video, described as “a text-conditional video generation system.” The idea is that we can make prime the system using a free text phrase such as “teddy bear skating in Times Square” or “tropical jungle in the snow,” and it will create 128 frames of 720p video at 24fps, a bit more than five seconds’ worth, featuring a subject with the absolute characteristics of the request. Perhaps disappointingly, it won’t return a picture of a robot holding up a sign reading “you want what?” in response to particularly abstruse requests, although all kidding aside that’s actually one of the problems with it, of which more below.
Generative Pre-trained Transformer 3
Creating still images based on natural language input is not an entirely new idea. Anyone who’s been keeping up over the last year will be tempted to relate Google’s work to the second version of OpenAI’s Dall-E, based on a technique described, with the opaqueness typical of cutting-edge AI research, as Generative Pre-trained Transformer 3, a process which was originally designed to create text output. The prose generated by GPT-3 is so good, apparently, that it’s hard to tell from writing created by a human being. Mumble, mumble, robot overlords, but the prospect is already creating concern over academic fraud and provoking the development of software designed to detect it.
Dall-E modifies that approach – whatever that approach actually is – to create images. If the oft-misquoted Einstein-Feynman conjecture about being able to describe things simply is right, GPT-3 is not well understood, because trying to elucidate how it works in a few sentences quickly leads down a rabbit hole, or a depthless, crushing rabbit black-hole, of esoteric AI terminology. It uses cascaded diffusion models and the underlying transformer deep learning model relies on self-attention, prioritising things which are of relevance while overlooking things which are not. And, er, 1-hot dictionary vectors and recurrent neural network encoders and…
To put this another way, Einstein’s ideas on special relativity are complicated, though the mass-energy equivalence E=mc2 really isn’t. Much of what goes on in AI, at least as far as neural networks go, is actually fairly understandable, but there are now so many tiers, each with its own jargon encapsulating yet more complexities, that even skimming the surface of how something like Dall-E works is way, way beyond the scope of an article like this.
The results speak for themselves, though the researchers show a degree of self-awareness in (almost) naming their creation after everyone’s favourite melting-clock enthusiast. AI-generated images are already establishing a reputation for creating images which range from acceptable, through vaguely unsettling, to downright eldritch. Similar problems attend Imagen videos, which show an elephant which looks fantastic at first glance – look, its ears flap in the breeze! - but whose front legs swap sides with each pace. Like a lot of AI mistakes, this glitch is made all the more unsettling by the combination of a physical impossibility with such a plausible, near-photographic rendering.
Similar problems attend requests such as “a teddy bear doing the dishes,” which does yield a video of a teddy bear doing the dishes, but on closer inspection shows the unfortunate creature’s paw occasionally becoming one with the dish it’s doing. The thing clearly has some understanding of what it’s drawing, but that understanding has limits, which is another problem: AIs don’t really know who they’re talking to, and they certainly don’t understand smutty double entendres, which creates certain risks given that someone who’s twelve might start asking about a pair of melons, with or without a snickering pre-teen’s understanding of what might result.
Ethical questions
This is one motivation that the Imagen people state for not having released their software. There are multiple cautionary tales of AI foulups, from Twitter bots spewing out hate speech that have to be turned off, to camera tracking technology focussing on a bald linesman’s head instead of the soccer ball at a football match. And if those two ends of the spectrum sound like comparing apples and oranges, they’re not. While one might be amusing and the other horrific, both display the problems that can occur when data sets are incomplete or biased. The AI itself does not have a leaning towards misogynistic behaviour or bald-shaming match officials, it is just processing data. The reactions and accusations are ours alone.
Given the fickle nature of human communication, it’s impossible to reliably predict every circumstance in which a system like this might cause political ructions or social embarrassment. Even then, considering real humans are capable of making faux pas in almost any given circumstance despite the benefit of a lifetime’s worth of experience, it’s a very difficult problem to solve, and even more difficult to prove to be solved.
Careful consideration of the training data sets are only likely to be a partial solution given their size and complexity, and the lack of any very accurate predictive metric for detecting problems in that data set. In the end it may be necessary for society at large to develop a healthy understanding that these systems ultimately lack the true intellect to be any more responsible for their mistakes than a below-average labradoodle. If we want AI, this is the compromise.
AI = Accelerating Images
What’s really impressive about Imagen is the speed with which it has emerged. Dall-E 2 was released in April this year, and Imagen in May. Imagen became capable of video this month. The challenges of temporal coherence – that is, avoiding flicker – have been tricky; things are still not perfect, and there are some shortcuts to be aware of. Imagen Video actually starts with 16-by-40 pixel video at three frames per second, and scales it up (a lot) in temporal and spatial resolution using preexisting techniques. That explains the slightly painterly look of the images, though the interpolation alone is hugely impressive.
Still, the overwhelming reaction from those in the AI community seems to be surprise at the pace of change. If we’ve got this far in this amount of time, whatever pressures AI is going to exert on society are likely to arrive much sooner than anyone thought.
Tags: Technology AI text to image
Comments