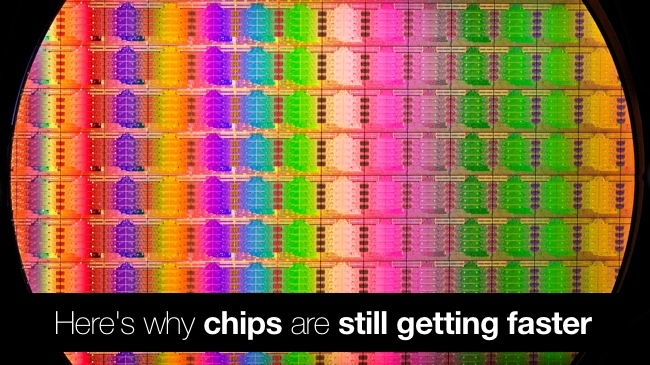
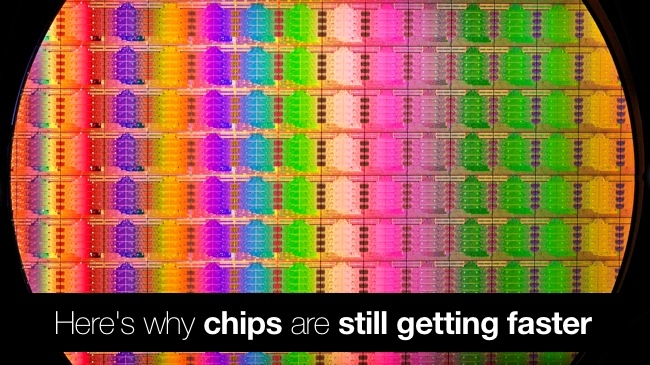
Guest author Rakesh Malik explores why we haven't seen great increases in processor speeds as of late, and how processors continue to improve in other ways.
Throughout the 90's and up until nearly 2010, it seemed like x86 processors were getting speed bumps every few months, with big jumps in maximum clock speed with every new generation.
Clearly, that's no longer the case. Clock speeds haven't actually gone up significantly for quite a while now.
This, of course, leads quite a few people to wonder, "why did the speed race stop?"
The short version is that it hasn't truly stopped; rather, it's changed. Clock speed isn't the only factor that determines the performance of a processor.
Pipelining
Pipelining is splitting the stages of execution into smaller stages, allowing for higher clock speeds.
To understand how this works, imagine a bucket brigade. If there are a few people in the bucket bridge, then each person has to walk back and forth to grab a bucket from the previous person and hand it off to the next. The walking back and forth makes each stage take longer, which in processor parlance is called latency. Making each stage simpler enables a higher clock speed. Imagine adding enough people to the bucket brigade so that a person can grab a bucket and hand it off to the next person without needing to even take a step.
The tradeoff is that if one of those stages gets stalled, all of them do. So if one stage is waiting on a bucket, everyone before has to pause. (We'll get to branching prediction later.)
Super-scalar
Most modern processors have a number of pipelines that can execute instructions in parallel. They're generally optimized for certain types of tasks, such as integer math or floating point math, ALU, and load/store. Issuing instructions in parallel is basically like having multiple bucket brigades working together, but there's a limit as to how many instructions the processor can issue at the same time.
Having a lot of execution units is one thing, keeping the occupied is another. The scheduler has to determine the most efficient way to schedule instructions of different types. It has to schedule them to retrieve data that instructions are going to need, accounting for how long it will take to load the data from memory into the caches and from the caches into the appropriate registers.
Pipelines that aren't executing instructions are just consuming power, and as the processors issue rate gets wider, it's also harder for the scheduler to keep the units busy. Because of this, the issue width of processors tends to cap out at a six-to-eight issue super-scalar.
Instruction Decode
Compilers generate instructions based on an Instruction Set Architecture, or ISA. Since that's a specification that spans a variety of processors, each version of the processor has to decode the compiler instructions into processor native instructions. In some processor architectures, each pipeline has a decoder, and some have just one decoder that saves the decoded instructions in a trace cache.
With a trace cache, the decoder doesn't have to decode instructions every time it encounters them, just the first time. This can add up to a significant improvement in efficiency and performance in programs that execute the same sequences of instructions repeatedly on a large set of data, for example.
Out of Order Execution
The instruction scheduler in the processor tries to keep as many pipelines and execution units as busy as it can. To do this, it will go so far as to figure out what instructions depend on the outputs from other instructions, and reorder them to keep the processor as busy as possible. The processor will also attempt to predict what data the instructions will need in order to complete executing, and load the data from memory to have it available when the instruction is ready to execute. If its rediction is correct, the processor stays busier, and if not then it suffers a mispredict penalty.
Some processors can issue instructions out of order, but must retire them in order. Others can issue and retire instructions out of order, which allows the scheduler to have additional flexibility in how to reorder instructions.
SIMD
Single Instruction, Multiple Data or SIMD is a way of packing multiple operations into a single instruction. Also known as vector computing, this is a common technique in supercomputers that is now ubiquitous in personal computers. Each vector contains multiple pieces of data, and the processor can execute that one instruction on all of them at the same time. One example is brightening all of the color values of one pixel in Photoshop by an integer value. This method involves packing the initial values into one vector, and adding that number to each pixel into another vector, and issuing a vector add instruction. Then all of the values in each vector are summed with one instruction.
This is requires explicit parallelization when writing the program in order to work, and some workloads are more amenable to parallelism of this type than others. Photo and video editing applications lend themselves to this sort of instruction-level parallelism quite well. 3D rendering applications generally do not, because their data access patterns are not very predictable.
Simultaneous Multithreading
Threads are similar to processes, except that they share their heap with their parent process and with each other. They retain their own program counter and stack, however.
For those who aren't familiar with these terms, the heap is where a program keeps its data, and the stack is where it tracks instructions.
A processor with simultaneous multithreading (aka HyperThreading in Intel-speak) can schedule instructions from more than one thread at the same time. That gives the scheduler some additional flexibility to issue instructions in parallel, which leads to keeping more of its pipelines busy.
Registers
The registers are where the processor's execution units get their data from and save it back to when executing instructions. If the data an execution unit needs is in the registers (where it's expecting to find it when it's executing its instructions), then it runs on unhindered. If the data isn't there yet, the execution unit has to wait for it, which in most cases stalls its entire pipeline. Modern processors have logic dedicated to predicting what data the processor will need and when, with the intention of loading it into registers before the processor needs it.
Cache
Hard disks have a very large latency for access, meaning that, from the time, the main processor sends a request for some data and the data that arrives is very large. So instead, processors load programs and data into main memory and execute from there. Main memory still has pretty high latency, particularly since it's typically running at clock speeds that can be 1/10 of the processor's clock speed.
To mitigate this latency, processors have high-speed caches on their dies. Some have one cache level, some have two or three. They usually have a small and first level cache that the processor uses to load data into registers, a second level cache that is larger but not quite as fast, and, in some cases, an even larger third level cache. In some processors, all of the cache levels are specific to a core, and in others the third level cache might be shared among the cores in a single chip, while the first and second level caches are dedicated to each core.
Multiple Cores
Multiple cores is basically the same as multiple processors, but on the same die. This approach reduces manufacturing costs overall since a single chip with six cores needs only one chip carrier. The interconnects between processors on the same die are also very fast, further improving parallel performance.
Clock Speed
Clock speed is an obvious way to increase a processor's performance. More clock cycles mean more work, but there's no free lunch. Increasing frequency increases power consumption, which increases heat.
In recent years, there has been a strong drive toward reducing power consumption in processors, while still improving performance. Thanks to the thermal wall, clock speeds haven't been increasing much.
New Technology
Developing ways to make their processors smaller allows manufacturers to put more transistors in a given sized die, and also enables higher clock speeds, since smaller transistors require smaller voltages to make them work.
It also leads to other difficulties, because the artifacts on processors today are so small that quantum effects introduce manufacturing challenges. One big one is a result of how traces are created on a wafer's surface.
The wafer has a substrate on it which is acid sensitive, until it's heated. To do this, a laser controlled by a precision stepper motor is passed over a mask. The mask works like a stencil so that that the laser is only cooking the parts of the substrate that are to stay. With the artifact sizes involved in modern lithography, diffraction through the mask can be pretty significant, leading manufacturers to develop techniques for controlling the laser's beam. For example, AMD developed a system of immersion lithography that puts a tiny droplet of heavy water in the space between the laser and the mask, and after one pulse sucks it back up, to put it in front of the laser after it's moved to the next spot.
After the laser does its thing, the wafer is washed in acid, removing the uncooked substrate. Then the next layer is vapor-deposited onto the wafer, and the process continues with the next layer.
Trade-offs and Innovation
As is typically the case, there are trade-offs with all of these approaches to improving performance. Increasing clock speed fell out of favor because of heat and power consumption. Adding pipelines can increase performance, but keeping the pipelines busy gets more difficult the more pipelines the processor has.
Increasing the pipeline depth enables the designers to increase the clock speed, but also increases the penalties for cache misses and for incorrect predictions about what data to load when.
Anything that adds logic to a processor's die increases its cost in two ways. One is that with a bigger die and a constant wafer size, there's room for fewer parts in the same surface area. Another is that even modern wafers have defects, and the probability of having a defect in a given part of a wafer is pretty much constant. Even though it's low, it's enough to cause fatal flaws in some of the dies. Since the probability of having a defect in the wafer is constant, a bigger die has a bigger chance of including a spot with a defect.
If the defect is in a cache block, then it's possible to work around it by remapping the cache block. Memory cells are relatively simple and relatively small in terms of die area, and therefore add relatively little to the cost of manufacturing, so it's feasible to design extra cache into a processor to increase yields this way. With logic however, a defect tends to do-in the processor.
When a wafer comes off of the line, it's cut into dies, and each die is tested at the chip's target clock speed, with a margin to ensure reliability. If it fails, it gets tested at the next clock speed tier down, until it passes. When it passes, it's put in a bin according to what speed it is reliable at. This process is called binning.
The marketing and business management staff figure out what the best distribution of processors is to maximize profits, since a processor can be marked for any clock speed below its rating, but not higher. The bulk of sales come from the lower bins, but the premiums that people are willing to pay for higher binned processors leads to high profit margins. This is why some processors from the same line overclock better than others, and also why unlocked processors carry a premium price tag.
To improve performance and power consumption, processor designers have to balance these methods, as well as develop new methods in order to continue improving performance. For a time, it seemed like clock speeds were the bee's knees...until the Pentium 4 hit the thermal wall and AMD managed to get the performance crown in some areas for a while. Now, power consumption is becoming an evermore important issue, and processor designers are striving to extract as much performance per watt as they can. Data centers and render farms are huge power consumers, particularly large ones with thousands of processors, so minimizing power consumption can make a huge difference in energy consumption and cost over time. The rise of the tablet as a computing platform also continues to drive increasing demand for processors that consume less power, yet there is a continued demand for more performance.
For most users, a modern Core i7 processor with two cores has more performance than they will ever need or use. For that matter, even the Core i3 processor in an entry level Surface 3 Pro or a dual-core ARM processor, like the one in the iPad Air, is more than enough for most casual computer users.
Single-threaded performance still matters for some applications, especially games, and similar computing applications that don't parallelize easily. Server workloads generally involve more parallel workloads, such as web and database servers, so processors made for servers generally trade-off clock speed in favor of additional cores and larger on-die caches, all the better to feed the additional cores.
Video editing and color grading parallize well, making server processors a good choice for video edit workstations. A lot of 3D renderers also make good use of multiple cores. Some of these applications are also well suited to GPU computing as well, due to how well they parallelize.
While clock speeds are increasing quite slowly lately, processor performance is continuing to increase, though not with the leaps and bounds as seen during the late 90's and early 2000's. Driving down power consumption has become a high priority, and power management techniques have become extremely sophisticated, allowing processors to turn parts of the processors off when they're not in use, and to adjust the clock speed in response to both workload and temperature.
For most applications, adding cores offers little or no benefit. Applications that don't parallelize well don't benefit from additional cores, and even for applications that do parallelize well, it takes considerable engineering skill to exploit it, and few engineers have experience developing parallel or distributed applications.
In addition to the thermal wall, the personal computer market is giving way to the mobile market, where tablets and cell phones dominate. Since even an entry level processor is now more than enough for casual computer users, there's less and less incentive for no-holds-barred processor designs. Some companies are building data centers with ARM processors in order to keep power consumption down. With processors designed for handheld computing deices that are powerful enough for web and database servers, and GPUs powering supercomputing clusters, it's getting harder and harder to maintain a high profit margin with high end CPUs.
For the most part in the current market, the primary markets for the higher end processor models are limited to dedicated gamers and content creators. The same people looking for high end processors also favor high end GPUs for computing as well as for realtime 3D rendering.
Clock speeds will probably never again rise like they did during the Athlon/Pentium4 heyday, but we'll continue to see improvements in performance. It just won't be as easy to quantify, much like the difficulty in determining the image quality a sensor is capable of based only on its megapixel count.
Tags: Technology
Comments