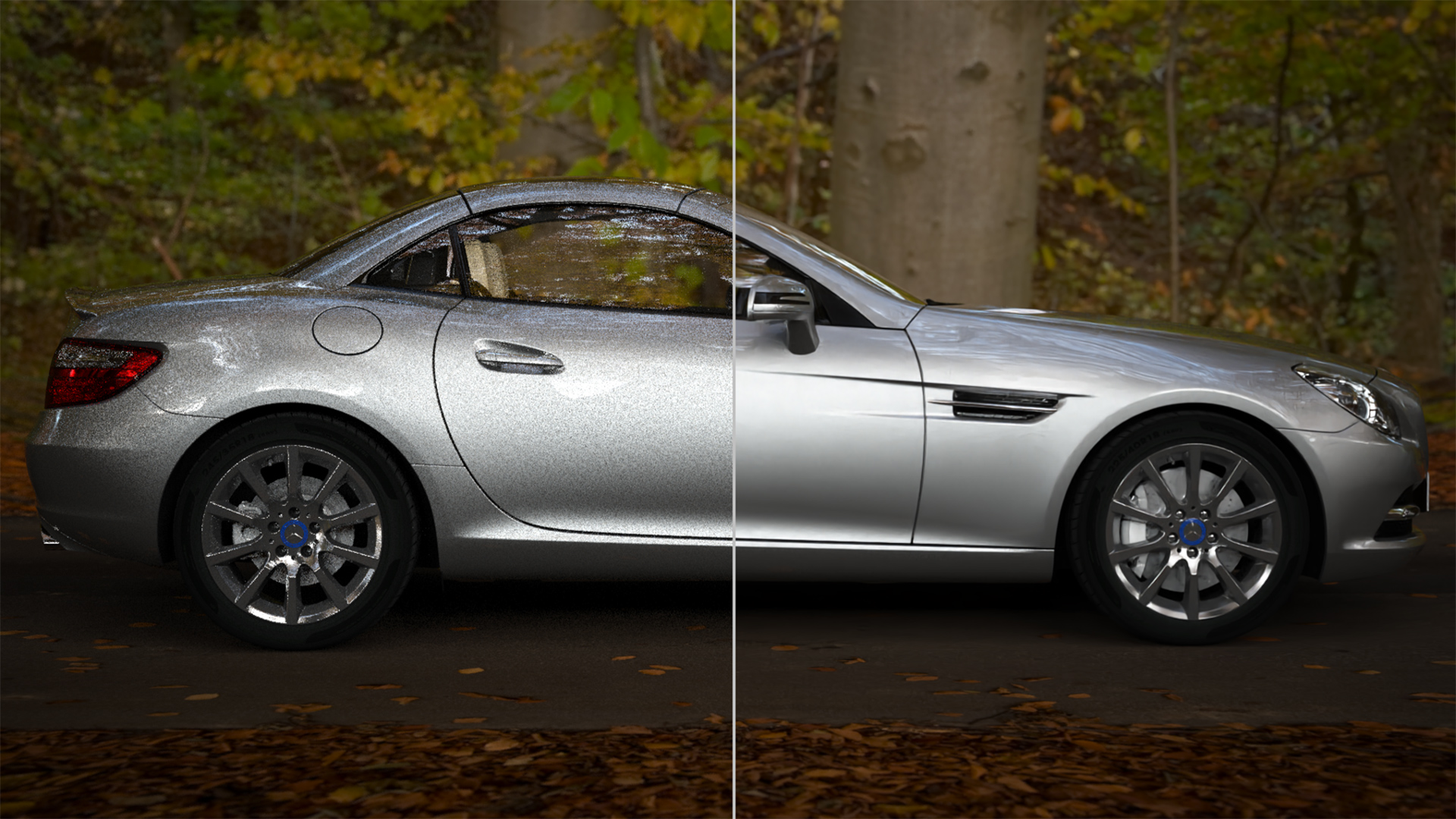
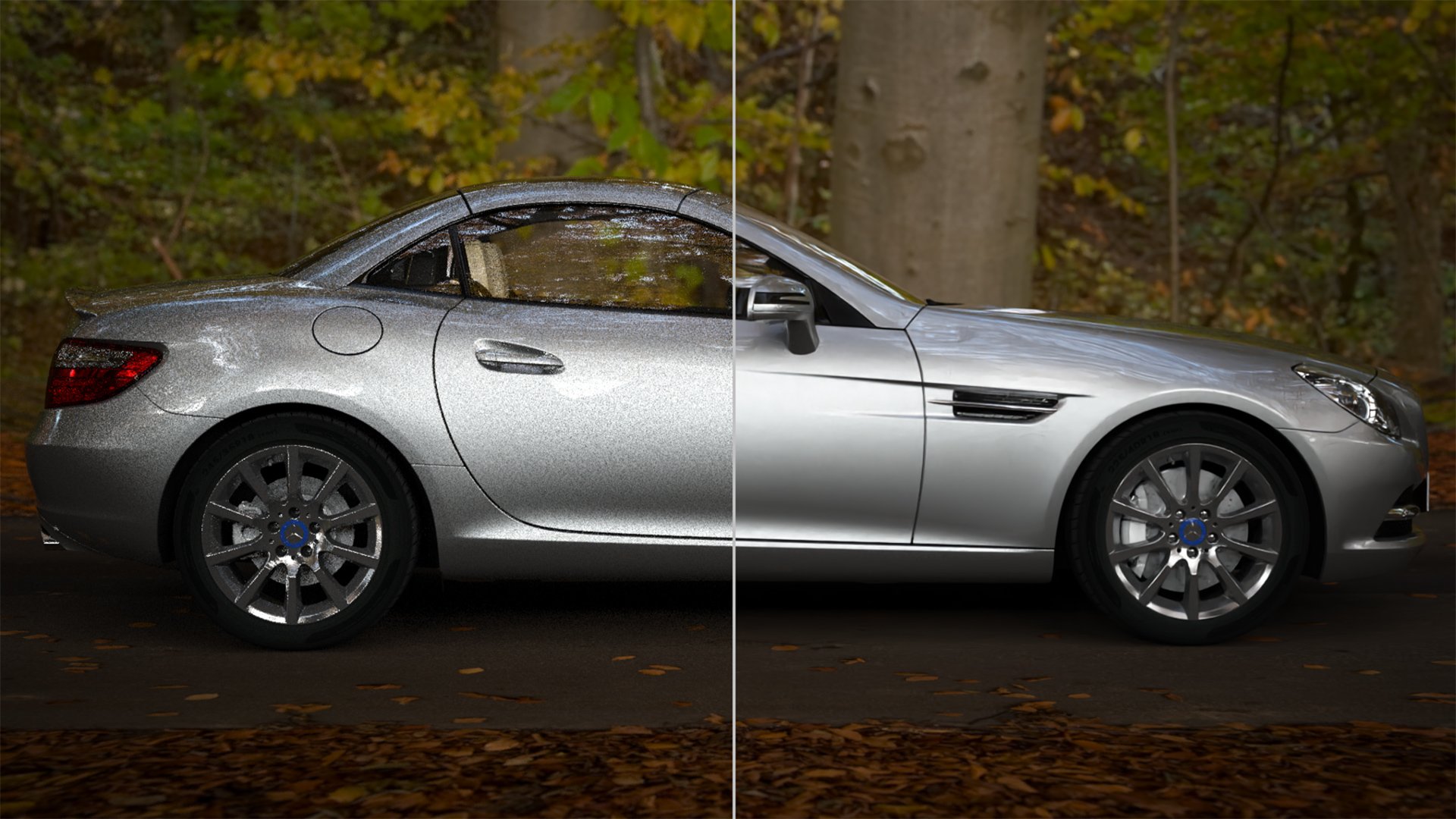
Nvidia wowed people last year with its real time AI assisted raytracing demos. But could similar AI techniques make real-time pixel-free video encoding a reality, too?
In many ways, 8K is similar to 4K. It's the same but times four. But while there's an easy case to make for 4K, and a similar, but admittedly harder, one to make for 8K: almost no-one is trying to convince anybody that except for scientific, security and perhaps immersive video, there's any need for 16K.
In which case, is this it? Does this mean that there's nothing beyond 8K? Can we all pack up and go home now, secure in the knowledge that the kit we are going to buy in the next few years is going to be current forever?
I think I know the answer, and I am pretty sure you're reading it for the first time here.
A few years back, I touched on this with this article (it's been republished since, but I think it first went out in 2013).
In it, I argued that we may never see 8K as a commercial, domestic format. Before that, we would have invented a new way to describe and encode video; one that used "vector" descriptions of the scene instead of pixels. It would be akin to Postscript or PDFs, which can be scaled to any size without losing resolution.
This is an imperfect analogy, though, because both Postscript and PDFs resort to reproducing bitmaps to describe complex images. Vector video would go beyond that and derive a vector description for every scene, and for motion within that scene too.
There are precedents, from the "autotrace" functions in graphics and drawing programs, and there's this genuine example of an attempt at vector video from 2012.
As you can see, it's only a proof of concept. There's an awful lot of work that needs to be done to make this real.
But once it's done, it would be watchable at any resolution and any framerate, because - just like with PostScript - it's the output rendering device (a screen, for video) that would determine these factors.
It didn't happen
Fast forward to today, and you can see that prima facie I was wrong. We did get 8K, and we didn't get vector video.
But look a little closer, and I'm beginning to think that I got it more right than even I thought at the time.
This is a big claim, and it could only possibly be true if there had been some huge changes since I wrote the first article. And there have been.
First of all, I just want to say that there's absolutely nothing wrong with my original ideas about vector video. All that was missing at the time was (probably) a thousand-fold increase in processor power, and a massive number of tweaks, optimisations and, yes, technology breakthroughs that would be needed to make it real.
But don't forget that while thousand fold increases in technology sound like they would take a long time to occur, that's exactly what happened with the iPhone.
The first iPhone came out in 2007. It was, again, little better than a proof of concept. It was the radical nature of the device that sold it - not its processing power.
But now, we have iPhones (and other smartphones, arguably even more powerful) that are on a par with mid-range Macbook Pros. They have the same high resolution screens, and incredible graphics and media processing. And they're handheld, and they run off a battery.
All things considered, it's not unreasonable to say that today's iPhones (and certainly tomorrow's) are a thousand times more powerful than the first one. That's in 12 years.
So, from that standpoint, you might want to say that if we wait another twelve years, we'll have the ability to do vector video.
But that's not only grossly oversimplifying things. It's just plain wrong, Because I think we're going to be able to do this next year.
The AI connection
The key to this surprising optimism is not Moore's law. We all know that physics is putting a damper on this empirical observation by the founder of Intel. It's been remarkably accurate until now, but the phenomenon is running out of steam. But faster than that, it's running out of relevance as well, because other technologies are overtaking it.
Foremost among these is AI, or, to be more specific, Machine Learning.
We hear a lot about this these days. It's everywhere, seemingly. It's rare to see a technology product launched without some reference to AI or ML. This is compounded by the fact that global web-services platforms from Google, Amazon and so on use AI too, so merely being a customer of those services makes developers, manufacturers, and brands think it's OK to say they're using AI.
Not everyone is as loose with the reality: some are genuinely using AI and ML for ground-breaking improvements in technology. It's just that right now, it's seen as a way to get ahead of competitors, even though it's dawning on the marketing people that if everyone's doing it, it's no so much of a commercial advantage, unless there genuinely is something novel in a product because of the new techniques.
I believe that AI is going to play a central part in new video formats. It's going to be able to do this because of the incredible developments we've seen over the last four years, and particularly over the last year.
How's it going to do this? It's a deeply technical subject, but I'm not going to do down a geeky rabbit hole. The things that matter are easy enough to understand - although sometimes hard to believe!
Examples
There are two examples of AI that I'll give that point towards the future of video formats. The first is Nvidia's remarkable announcement in August 2018 that its new GPU architecture, "Turing", would be capable of real time Ray Tracing.
This was remarkable in itself, because until August, if you'd asked anyone - anyone - outside of Nvidia when real time ray tracing would be possible, the unanimous answer would have been "not for at least ten years". Now, it's not only possible but you can buy the parts you need in a shop for the sort of budget that makes nobody flinch.
The new GPUs have AI accelerated features that essentially watch a render as it's being completed. At some point - long before it's anything like finished to full resolution - the AI will say "I know that that is: it's a class of water" or "a steering wheel" or "an armadillo", and it will use what it has learned (or been taught) to complete the object to the full resolution of the final render.
Since it's usually the fine detail that takes the most time in rendering, this represents a huge breakthrough and a massive advance in speed. Fast enough, in fact, to complete an entire image in less than the duration of a video frame.
Can you see where this is heading? I'll come back to this in a minute.
Describing a scene
If you're going to move beyond pixels, you have to find an alternative way to describe a scene. Compression codecs do it all the time, some of them looking at the entire scene at a time, like wavelet compression; some of it looking at smaller blocks of pixels. Long GOP codecs analyse movement within a scene as a means to reduce the amount of data needed to describe a sequence of frames. Part of this is "vectorising" motion within the scene.
For several decades, computer drawing programs like Adobe Illustrator (and the venerable Coral Draw) have used outlines and fills to describe images. Pictures are only turned back into pixels for viewing on a screen, or for printing.
Typefaces are commonly stored as vector descriptions, not bitmaps. That's why they look good at any size - and why you can zoom into a PDF document and no matter how big the letters are, you won't see any aliasing (jagged edges).
Wouldn't it be great if you could do this with video? Imagine being able to scale video to any size with no apparent aliasing?
You can't simply wave a magic want and make this happen. There's some hard work to get there. In the five years since my original article, nothing much has happened to suggest that we've made any progress here, and that's why 8K has stepped in: because there's nothing radically new on the horizon.
But I think there now is something radically new. So much so that we can only guess at what it means (and please understand that this is only at best a complete guess - but I think it's a reasonable one).
I believe that AI technology is going to play an intrinsic part in future video formats. I'm going to restrict what I say here to "conventional" video: the sort we watch on a screen.
You've probably seen thispersondoesnotexist.com.
It's a simple website designed to demonstrate the rather staggering reality that AI can now generate pictures of people who have never existed, and which look so good, that a casual viewer wouldn't suspect that they are computer-generated. It doesn't matter how often you refresh the page: you won't see the same image twice. That's because they're all generated on the spot. They're unique.
They're not perfect. You can often spot weirdness. But the reality is that they're often completely convincing in the sense that a casual viewer wouldn't think twice. In this sense, it passes a kind of Turing test.
What's remarkable is that the system seems to have no difficulty in generating hair, eyes, skin textures and blemishes. Lighting and shadows look utterly convincing.
The AI in question has been trained using thousands of pictures of people of every size and shape. It "knows" what eyes, noses, ears and skin look like. But what exactly is this "knowledge"? Are we making mistake when we talk about it in such anthropomorphic terms? That's hard to answer. But while it's a good question, it's not the point here.
What is the point is that the AI that created these pictures doesn't think in terms of outlines and fills. It doesn't even consider textures as distinct from objects and surfaces.
It certainly doesn't try to fit its observations into man-made or even any sort of pre-existing structure or taxonomy. We actually don't know what it does, or how it does it.
And that's the strength of it. Because it's not bounded by the restrictions that we would impose on it if we were going to write a hard-coded program to generate new faces.
No preconceptions
This factor is so important that it's hard to take in. I'll try to explain it. The key is that with artificial intelligence, we're not just limited to things that we can think to describe a picture (outline, colour, brightness etc). AI has none of these preconceptions. When an AI system is in the process of "learning" - or perhaps "training" would be a less anthropomorphic word - it looks for patterns. This doesn't just mean patterns of colours, or shapes, or textures, it means absolutely anything about one image that it might have in common with others.
Let me try to illustrate how important this is. (I know this is taking a long time to read, but this is so important to the concept)
Have you ever wondered what it means when you say someone resembles someone else? We say that someone has a family resemblance if - for any reason - you can tell they're related to someone else. That's pretty easy. You may even be able to pin it down to the shape of a nose, or eye colour.
But what happens when you see someone else that you also think is related, but they have a completely different nose, or eyes, or anything else?
The specific problem is that you may be able to recognise resemblances, but be unable to be specific about all of them. The fact is that the things that mean you can recognise one person as a family member may not the the same as the factors that make you recognise someone else. In fact, it's possible that you can identify one person as a family member and for them to share absolutely none of the characteristics of another person that you also identify as a family member.
This is not a new observation. The 20th Century philosopher Ludwig Wittgenstein wrote about this in a book published in 1953, after his death, called, somewhat generically, Philosophical Investigations.
It's a serious problem, especially if you want to put things into neat categories.
But - as we are becoming increasingly aware - it's not a problem for AI. AI doesn't bother with pre-made categories: it makes up its own, based on what it "sees" during its training. And the effect is both remarkable and life-changing.
Because in devising its own categories and hierarchies (taxonomies) an AI can go where we don't, bound, as we are, by our own preconceptions. Here's an illustration. We tend to be constrained by categories and dimensions that we're familiar with. Time, space, geometry etc. I'm not going to say that AI is a time and space machine - this is just an illustration - but what it can do is find connections and similarities that we don't even have words for. Think of it as being able to use maybe 17 dimensions instead of three. It really is that big a difference. (I don't mean 17 dimensions of space and time, but 17 - or any number - of scaleable factors: call them "vectors" if you like.)
And, having found these crucial distinctions and resemblances that would never even have occurred to us, it can use this finely-tuned ability to be incredibly accurate with its selections, in ways that we might not understand.
Coming at this from the opposite direction, An AI with this "knowledge" can use it to create images that have never existed. If we tried to do this, we would be stuck with our old conceptual framework. Our creations - stuck in our limited and constrained dimensionality - would seem weak and clumsy.
Thispersondoesnotexist.com is the perfect example of this. It makes faces not just recognisably faces, but uncannily real, and all the more so because they have never actually existed,
So, how does this apply to video?
Let's imagine that we have a system that we have trained on millions of images. The knowledge that’s been acquired can be encapsulated and stored in a relatively small space. Video in a camera can be turned into a multi-dimensional vector format by the AI. It will look almost perfect at any resolution. Any artefacts can be corrected by the “knowledge” of what an object or texture “should” look like. You can see from the
“Thispersondoesnotexist” website that images can be made to look very convincing. Just imagine what they will look like when they’re based on reality.
The point is that these AI-constructed images are not based on pixels: they’re based on concepts and - essentially - vectors representing relationships and similarities. They are not bounded by our own categories but by patterns and resemblances that the AI itself has discerned.
Does all this sound a bit wild? Maybe a bit too theoretical? I would agree with you. But at the point where an AI system can create convincing images of people who have never existed - and good enough to fool almost anyone, and at the point where this technology is improving almost by the second - it’s hard to envisage that it won’t form the basis of some future video format
Tags: Technology
Comments