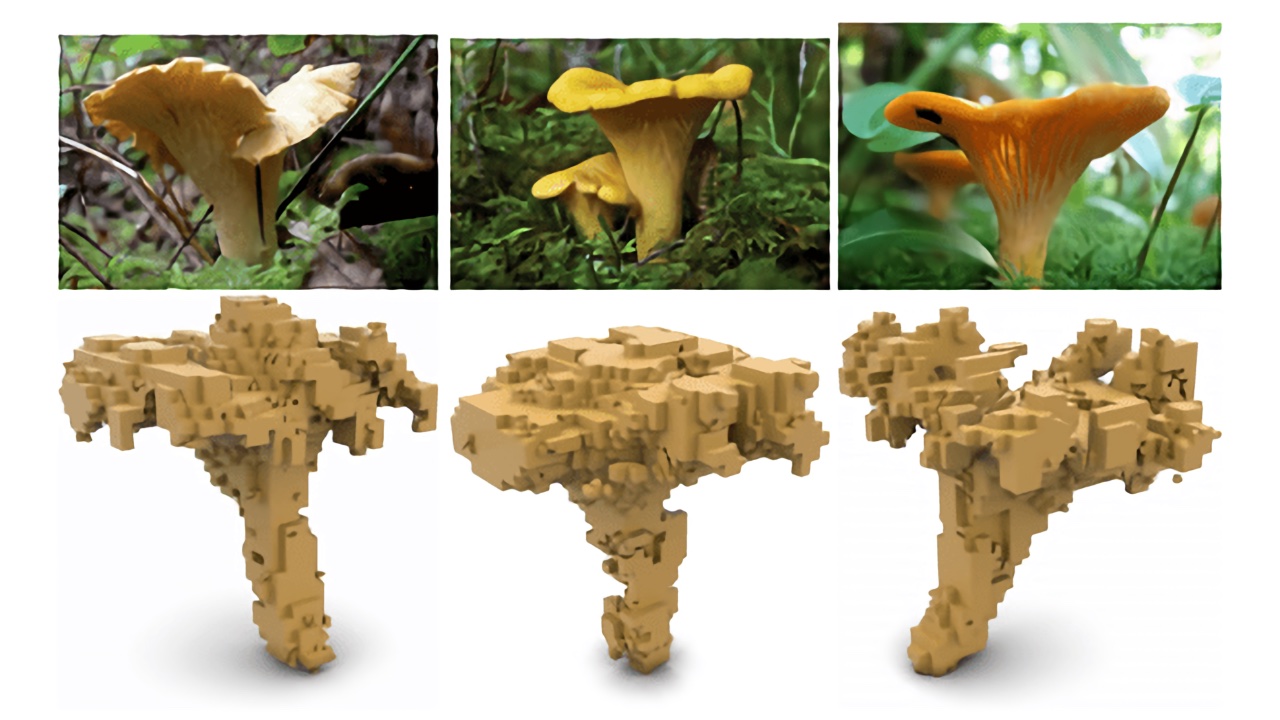
Researchers have written an algorithm to derive 3D graphics from 2D data, quickly and at scale
Microsoft researchers claim to have devised an AI able to generate better 3D shapes from 2D images and to do so for the first time using off-the-shelf photo-realistic renderers like Unreal Engine and Unity. The result could help make video games or animated content production cheaper and quicker.
A recent research paper introduces what is described as the first scalable training technique for 3D generative models from 2D data.
While Generative Adversarial Networks (GANS) have produced impressive results on 2D image data, many visual applications, such as gaming, require 3D models as inputs instead of just images.
GANs are two-part AI models comprising of generators that produce synthetic examples from random noise sampled from a distribution, which along with real examples from a training data set are fed to the discriminator, which attempts to distinguish between the two.
Training data
Since directly extending existing GAN models to 3D requires access to 3D training data, this data is expensive to generate. The researchers set out to build an AI that can learn to generate 3D models while training with only 2D image data, which is much more widely available, much cheaper and easier to obtain.
VentureBeat explains explains that, in experiments, the team employed a 3D convolutional GAN architecture for the generator. Drawing on a range of synthetic data sets generated from 3D models and a real-life data set, they synthesised images from different object categories, which they rendered from different viewpoints throughout the training process.
The researchers also used light exposure and shadow information in the rendering engine, to generate high-quality convex shapes, like bathtubs and couches, that previous attempts had failed to capture.
In theory, the technique can be extended by using more sophisticated photorealistic rendering engines, to be able to learn even more detailed information about the 3D world from images.
“By incorporating colour, material and lighting prediction into our model we hope to be able to extend it to work with more general real-world datasets,” they conclude, leaving others to pick up the ball.
Tags: Post & VFX
Comments