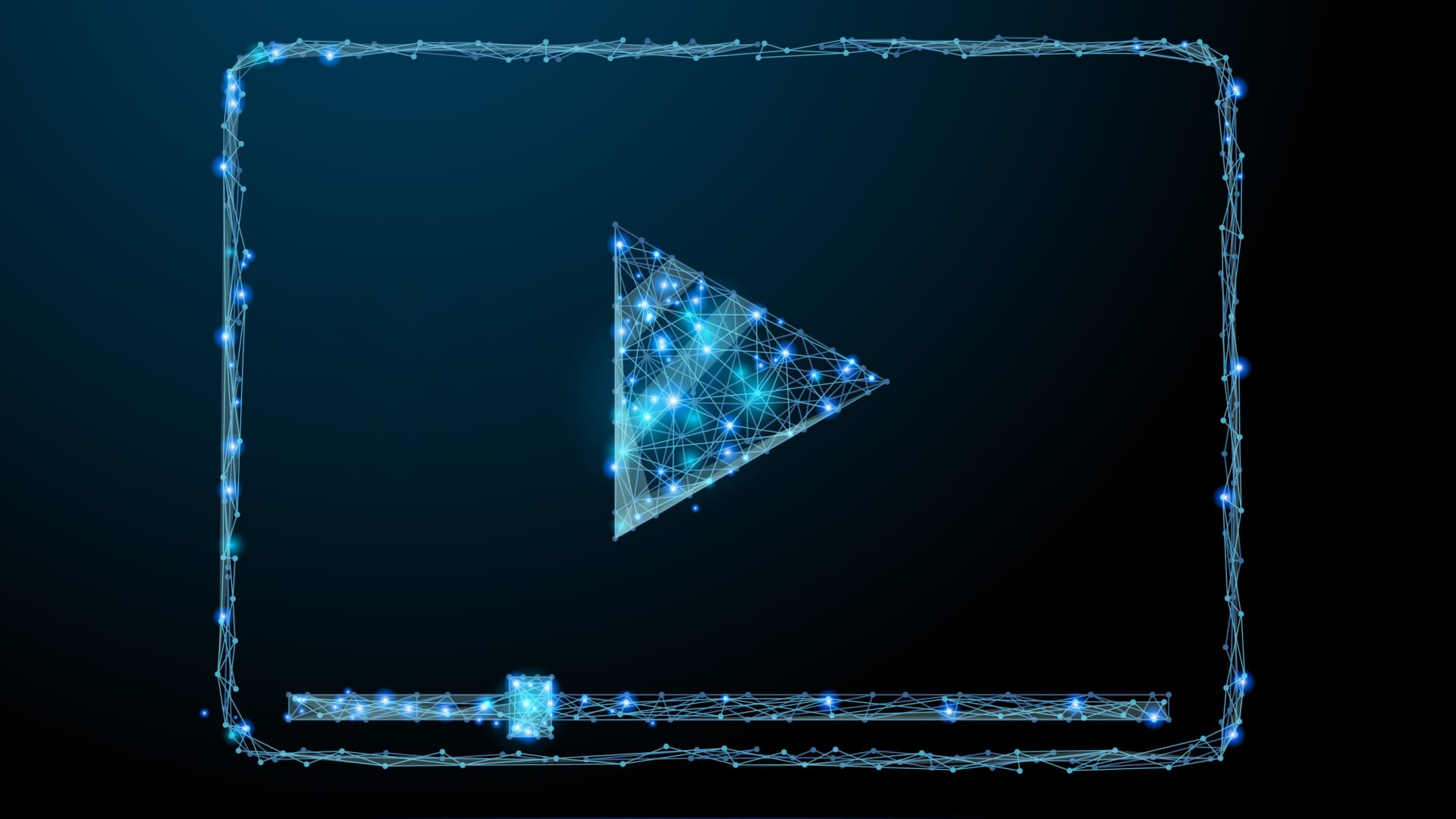
Away from all things generative for a moment, what would be the advantages of AI-encoded video and how would it work?
Ai is growing so fast it's easy to imagine it will be able to do anything. In one sense, that is almost inevitably true. When it becomes super-intelligent - and the start of that would be when it is as intelligent as us - it will be able to do anything that we can, including designing new technology. It's already happening in some limited ways: show a sloppy piece of Python code to GPT-4, and it will tidy it up. Imagine what it will do in ten years (or even one year). Nvidia, the company that builds the GPUs and dedicated AI chips that are fuelling much of the AI revolution, reckons that we've seen a million-fold increase in AI in the past decade and will see the same again in the next one. So that's a trillion-fold increase in just 20 years. I don't even know how to think about that. Hold on to your hats!
But what about encoding video? Conventional video compression uses mathematics to remove information that won't be missed. While it's imperfect, it can be remarkably efficient, and mild compression is invisible to most viewers.
So how would AI video compression work, and why would we want it?
Key advantages
Two key advantages would be that it wouldn't use pixels and it wouldn't divide video into frames. Instead, you can view AI video at any resolution and framerate. Let's see how this might work.
Ai has quite suddenly become good at recognising images. The newest models are now "Multi-modal" in that they can interpret images as well as text. I've seen reports that you can show GPT-4 your design for a website, literally sketched on the back of an envelope, and it will create that website for you in actual code. You can show a diffusion model a photo of your face, and it will redraw it in the style of Van Gogh, Rembrandt or David Hockney.
So what if you showed it a photo and told it to show you the same image in as high a resolution as possible? If it can make something up (albeit based on input from millions of existing images), it's not unreasonable to think that it could recreate a photo that you're holding up in front of it.
If you try this today, it doesn't work. It's looking in the wrong place: at its own training data, which, of course, doesn't contain your photo. At best, you'll get multiple versions of similar-looking faces. But it's likely to be possible to create models that will try to replicate an image that it's "looking at". Existing models already contain a lot of the necessary data. So it just needs to work at a much smaller level of detail.
So, for example, it would need to look at the texture of your skin and the hairs on the back of your hand rather than the level of the furniture arrangement in an imaginary living room. That shouldn't be too much of a problem because neural networks and their relatives are fundamentally hierarchical in that they work on the small details before building the bigger picture.
Instead of storing video as a grid-like array of colours, AI would capture concepts like the shape of someone's nose, their glasses, a pattern on their shirt, and that the picture was taken at sunset. It would also capture concepts that we wouldn't understand.
But would this even be desirable? What are the downsides?
Making it work
The biggest might be that it takes a lot of work to make it work convincingly and would require a vast amount of processing. That's not ideal for video. If it takes an hour to encode a single frame, it's not practical for a camera. But remember, with a million-fold increase in AI performance in ten years, that disadvantage may go away given a bit of time.
The same applies to the question of accuracy. When you understand how high-ratio compression works, you might struggle to believe it could reproduce an accurate picture. It's so reductive that what goes on at the lowest levels seems to have only a tenuous relationship with the original (or reproduced) image. But if you zoom out to a more macro level, all that complexity and abstraction fades into the background to reveal a remarkably accurate picture.
It's encouraging to see that even current diffusion models have a concept of "Photorealistic". Put this term in the prompt, and you're likely to see a glossy and crisp-looking result.
Remember that AI models don't store pixels any more than we do when we recall an image or scene from our memory.
One enormous remaining hurdle is making one frame follow on from another without looking like it was painted by another artist. If you ask an AI diffusion model to create a video, each frame will differ stylistically from the ones before and after. That's because the models have no concept of temporal continuity. But this is unlikely to be an issue in the long term. Temporal continuity is just one more concept that the model needs to learn. Well, perhaps that's an oversimplification. It might require a new model from scratch, but that's hardly difficult these days, or especially in the future.
With today's technology, none of this would work well enough to be useful. But if you're prepared to wait three weeks or so...
Tags: Technology AI
Comments