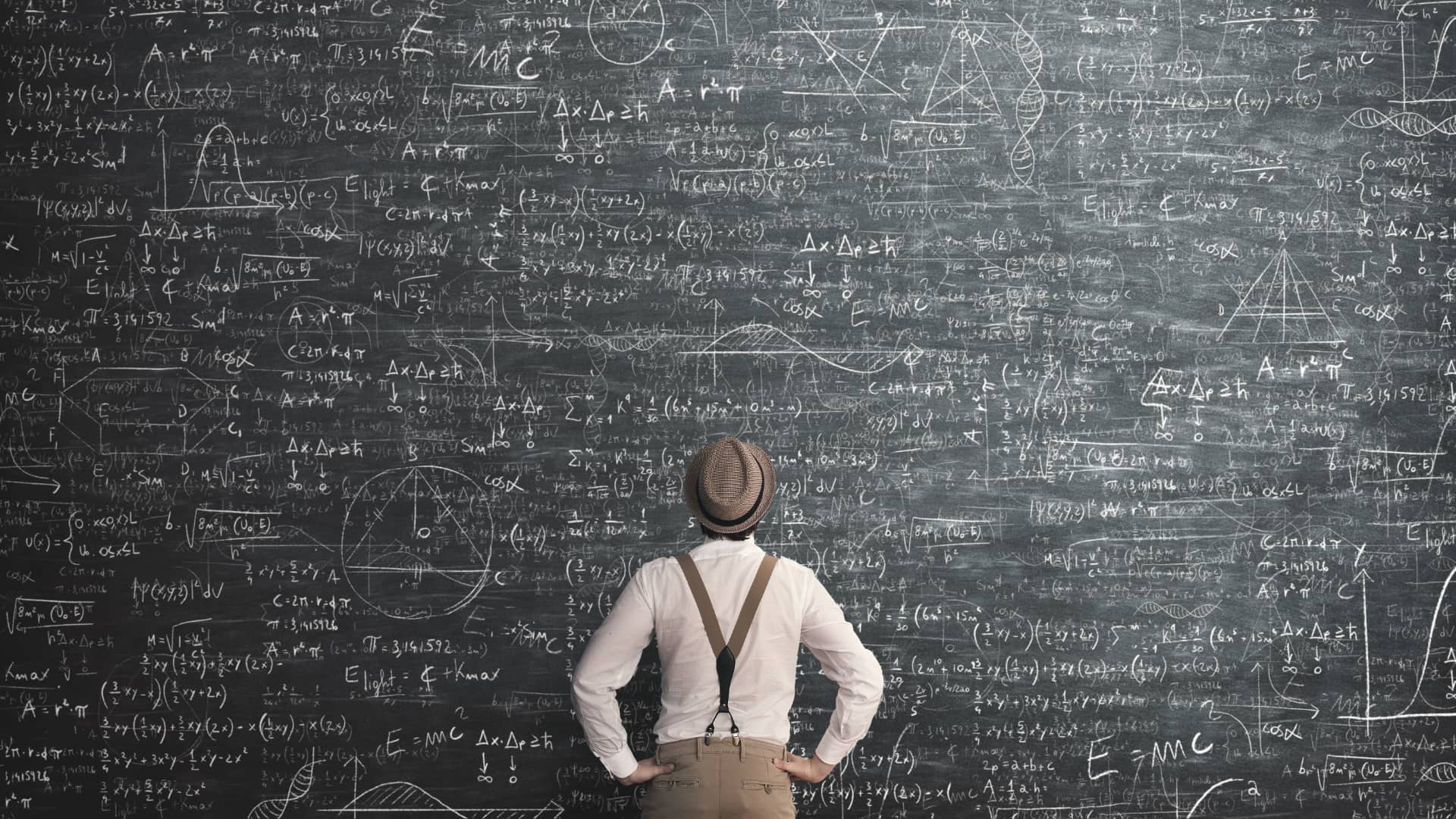
There's something about AI (Artificial Intelligence/Machine Learning) that seems hard to grasp. Even engineers, programmers and scientists can find it a bit baffling. David Shapton is your guide to untangling the complexity.
Baffled by AI? That's OK. It's in the very nature of AI that it is somewhat transcendent, that it's removed from our everyday experience and expectations, that it's somehow "other-worldly". If you had to sum up the problem in the most basic language, it's this: it's hard to see how any combination of hardware and software can claim to have properties that have previously only been observed in complex, sentient beings.
Part of this is due to expectations and needing to understand the nature and degree of abstraction required for AI to exist. What do I mean by abstraction? Think about your laptop. How can it show you pictures of a beautiful flower, allow you to have video calls with your neighbour or a friend in Australia, or let you compose music with virtual instruments? These are all easily explained by understanding that you have layer upon layer of software, each of which takes you further away from the basic ones and zeros that are the currency of computer processing. Combinations of binary code can represent building blocks like letters of the alphabet, any number, however big or small, and vast arrays of numbers that can convey pictures. If those numbers can change fast enough, they can also represent video.
So why is AI so challenging? Don't worry. It's not just you. Read on to at least make a start on this journey.
The Bucket Brigade effect
Musicians of a certain age will remember that, in the early 80s, you could buy guitar effects pedals with an oddly-named chip called a Bucket Brigade Delay. This little component had seemingly miraculous properties. For example, you could feed an audio signal into it, and it would apply echoes and even reverberation. That seemed surprising because how on earth could you get reverb from a small black plastic solid-state chip? Previously artificial reverb required a mechanical component like a spring or a heavy metal plate. Actual reverb needed a cathedral. It seemed like it shouldn't be possible.
You can google an explanation in seconds, so I won't go into it here, but it was merely a clever application of lateral thinking and technology that wasn't massively sophisticated. These BBD chips were later replaced by fully digital equivalents, and now you can have an entire recording studio - complete with myriad effects - running on your phone, iPad or laptop. That, too, would seem impossible and surprising to anyone who had been asleep - or perhaps merely not paying attention - for the last decade or so.
I remember that feeling: a combination of "This is not what I would expect from this device" to "What have I missed...".
Today, this feeling is increasingly common. The more we see AI doing valuable things, the more we find ourselves thinking, "How is this possible?" which is accompanied by another feeling: "I don't understand this".
The most surprising thing is that you can describe something in a few words and get a photorealistic rendering of your idea. So how do the chips in your computer do this?
One clouder
To be fair, they don't. Most of the text-to-image services are processed in the cloud. Your computer could do it in principle, but it would either take ages or not be as good. And cloud services are better when hundreds of people are trying to do the same thing simultaneously. But those services are running on everyday, albeit powerful, computers with fast GPUs and perhaps dedicated AI chips in some cases. But still, it's all just hardware and software. None of that explains how that technology stack can paint a unique picture in response to a few words of instruction.
We often hear about "training" in the context of AI, which is pretty counterintuitive, because how do you "train" a bunch of transistors? For example, if I were to show a bunch of flowers to a CPU, precisely nothing useful would happen.
The answer is - again - found in the software and in the way that software layers interact with themselves.
Most of us have heard that neural nets are good at recognising patterns. We won't go into that mechanism here because there are plenty of explanations online. But it's much harder to see how you can get higher levels of so-called intelligent behaviour from merely spotting familiar patterns.
But that's exactly what our brains do.
The real world - the reality in front of us - doesn't come with labels. All we get is raw data. And sometimes it's not enough. You'll know the feeling if you've ever had to drive in pitch darkness without abundant visual information. It's easy to make mistakes.
But, somehow, we learn how to navigate through the world, deal with hazards, and seek out goals. Through our senses, we see colourful, realistic images, perceive high-resolution sounds, we taste and smell and use lesser-known senses like proprioception to judge and understand where our limbs and body are in relation to the framework of reality that we live in.
What's the equivalent of this kind of capability in AI? A realistic answer is that it doesn't exist yet, although there is astonishing progress in the field of Artificial Narrow Intelligence (ANI, as opposed to AGI - Artificial General Intelligence).
The tricky thing about understanding AI is that there's a massive gap between our intuitive knowledge of circuits and software and the apparent ability of machines to "think". But machines don't "think" yet. It's just that they're starting to look like they might.
Here's how they'll think...
Through devices like Neural Nets, an AI system can "learn" what something - anything - is "like". That's pretty much in the same way as if a visually impaired person were to ask you what an elephant is "like" - you'd start by describing, perhaps, that it had four legs, was very big, and had a trunk. The sightless person's acquired impression of an elephant would represent what that animal was "like".
In the next article, we'll start to tie all this together and explain what makes AI grow faster than any technology we've seen before. In the AI community, we're starting to see comments like "I've been away for two weeks, and I'm astonished by what's happened in that time". There has never been a time like this. And this is just the start.
Tags: Technology AI
Comments